Significance of Machine Learning in Advanced Process Control
- picontrolsolutions
- Oct 26, 2023
- 3 min read
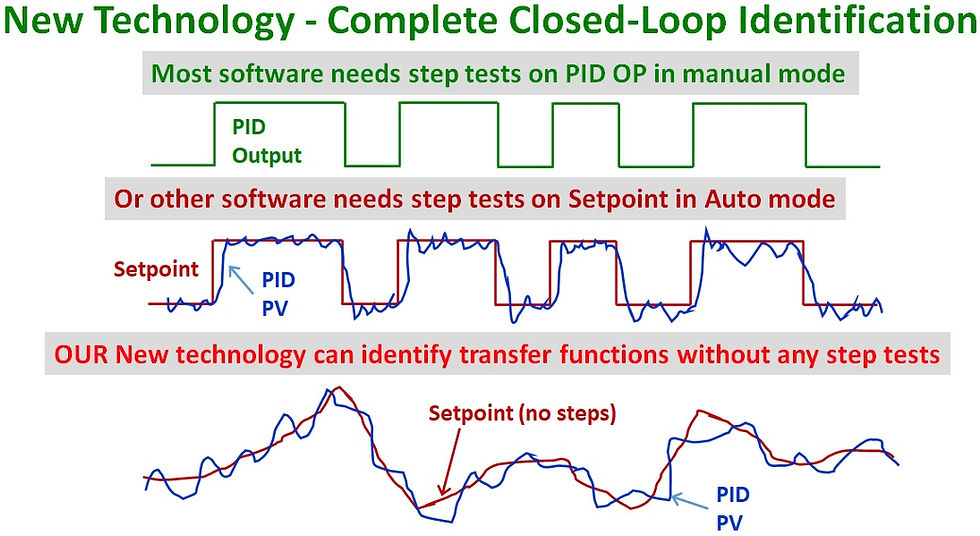
In today's fast-paced and data-driven industrial landscape, the need for advanced process control is more essential than ever. Industries are constantly seeking innovative strategies to make the most of their processes, cut down costs, and improve productivity. Machine learning, a subset of artificial intelligence, has come out as a game-changing technical concept in achieving these objectives. We will delve into the role of machine learning in advanced process control, exploring its applications, benefits, and the potential it holds for various industries.
What is Advanced Process Control?
Advanced process control (APC) is a procedure that employs real-time data and automation to enhance the output of complex processes. These processes find application in chemical manufacturing, energy production, semiconductor fabrication, and even autonomous vehicles. APC goes beyond conventional control methods by optimizing multiple variables simultaneously, resulting in superior product quality, reduced waste, and energy conservation.
Traditional control systems, like PID (Proportional-Integral-Derivative) controllers, work well for simple processes with few variables. However, they are not effective in managing complex systems with interconnected variables, where interactions can be nonlinear and unpredictable. This is where machine learning steps in.
Machine Learning in Advanced Process Control
Machine learning brings a data-driven, adaptive approach to advanced process control. It excels in handling the complexity and uncertainty associated with modern industrial processes. Here are some key applications and benefits of integrating machine learning into advanced process control :
Predictive Maintenance: Machine learning models can predict when devices are likely to fail, enabling proactive maintenance. This prevents costly downtime and maximizes equipment lifespan. In industries like manufacturing and energy, predictive maintenance is a game-changer.
Anomaly Detection: Machine learning algorithms can identify abnormal patterns or anomalies in process data, which could indicate a problem or inefficiency. By identifying these anomalies in real-time, processes can be adjusted to prevent defects and minimize waste.
Optimization: Machine learning models can continuously optimize process variables, taking into account both short-term and long-term objectives. This results in better resource allocation, reduced energy consumption, and improved product quality.
Complex Systems Control: In processes where multiple variables interact in complex ways, machine learning models can find optimal control strategies that traditional methods cannot. This is especially beneficial in industries like petrochemicals, where precise control of various parameters is crucial.
Dynamic Response: Machine learning can enhance the dynamic response of systems by adjusting to changing conditions. This is essential in applications like independent vehicles and robotics, where real-time decision-making is critical.
Quality Control: In manufacturing, machine learning can help maintain consistent product quality by adjusting process parameters based on sensor data. This reduces defects and waste, resulting in cost savings.
Energy Efficiency: Machine learning can optimize energy consumption in industrial processes by adjusting parameters in real-time. This has environmental benefits as well as economic advantages.
Customized Solutions: Machine learning allows for the development of customized control strategies tailored to a specific process, which can outperform generic control algorithms.
Real-World Applications
Machine learning in advanced process control is more than just a hypothetical idea; it's already making a significant impact in various industries. Here are some real-world examples:
Pharmaceutical Manufacturing: The pharmaceutical industry depends on rigorous quality control. Machine learning helps maximize processes and reduce the likelihood of faulty products, assuring patient safety.
Oil and Gas: In oil refineries, machine learning is used to enhance the productivity of distillation and cracking processes, leading to substantial energy savings.
Semiconductor Fabrication: In semiconductor manufacturing, machine learning is used for fault detection and process optimization, resulting in higher profits and lower production expenses.
Agriculture: Machine learning is used to make irrigation and fertilizer more effective, reducing resource use and environmental effect.
Financial Trading: In the world of finance, machine learning algorithms regulate high-frequency trading systems, making real-time decisions to optimize trading strategies.
In conclusion, the incorporation of machine learning into advanced process control is a transformative development in a wide range of industries. It enables businesses to enhance efficiency, reduce costs, and improve product quality while adapting to changing conditions and complexities.
As technology continues to evolve and more applications emerge, the role of machine learning in APC is set to become increasingly indispensable, reshaping the way industries approach process optimization and automation. Visit PiControl Solutions LLC for robust, compact and self-contained advanced process control software and make your business more productive.
Comments